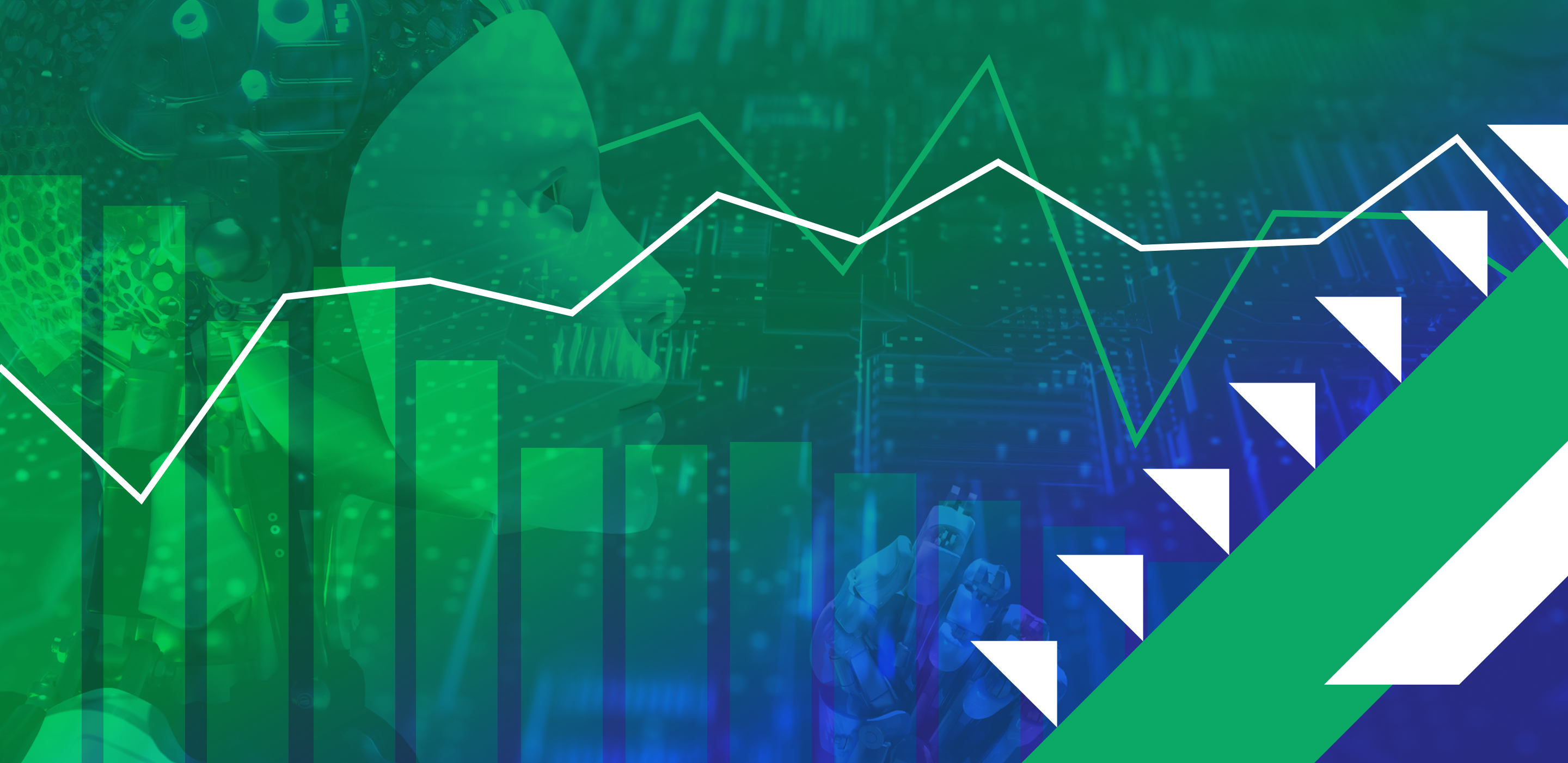
Aug 28, 2023
Tell Me How You Really Feel: Sentiment Analysis and Its Role in Customer Support
digital transformation
From tweets to text messages, the words we write have meaning. That meaning, however, isn’t always obvious to the reader. That’s what makes sentiment analysis technology so powerful and promising, with around 53% of companies using it today.
While customers are more vocal than ever before, it’s not hard to misinterpret what they mean. Sentiment analysis uses technology to read between the lines, anticipating the needs of customers based on how they feel about you, your product, or their customer experience. It enables you to head off problems and even solve issues customers don’t realize they have.
What is sentiment analysis?
Sentiment analysis is the process of using natural language processing (NLP) and machine learning algorithms to scan and analyze a piece of written text to determine the sentiment behind it, even if it’s not explicitly communicated. Generally, the result isn’t binary (good or bad) but can include emotions we associate as positive sentiment (excited, happy, or thankful), negative sentiment (frustrated, confused, or angry), or even neutral (factual statements).
Customer sentiment analysis is a specific application of this technology that automates the scanning, processing, and measuring of text for insights that can help customer support teams do their jobs better. The analysis is used in communications both to and from the customer.
This technology works best when created from a large, historical body of data across all customer cases and interactions and then applied to an individual customer journey. While the machine learning models are built on what’s worked for many customers, what may be frustrating or confusing for one customer may not be for another. Humans are still needed to apply these insights in personal interactions with each customer.
How sentiment analysis works
This technology can be very effective because it makes use of every interaction with customers to build a large library of data. However, each customer case can be managed uniquely.
Sentiment analysis can determine if the customer is upset about the product and requires help from product support or development to get the answer they need or if they are frustrated with their customer service experience. In this second example, insights may tell you that it’s a process or training issue that can have a big impact across the organization.
“With SupportLogic’s technology, you don’t need to worry about communicating customer support improvement opportunities across silos, and we can get this information in a timely manner,” Charles Monnett, the director of product management at SupportLogic, explains.
“We can address these things without waiting six months to get an NPS survey or waiting for the customer to churn. We don’t have to look around at each other and say, ‘Well, what happened there?’ Getting that sentiment analysis in real time can really help you be more proactive and prevent these kinds of situations.”
No matter the reason for a customer support interaction, sentiment analysis can drive faster case resolutions.
4 use cases for sentiment analysis in the customer support experience
Where can you use this technology? There are more than a few areas of opportunity.
1. Self-service tools
Automated customer service and self-service tools are very popular, but these conversations happen very quickly. With sentiment analysis built into your chatbot workflows, it’s possible to detect when a customer case should be escalated to a human agent, reducing the frustration that comes from “arguing with a robot.”
Customer sentiment analysis also gives you a layer of observability over self-service tools, allowing chatbots to work efficiently while you collect insights and signals from the conversations. If your chatbot has 400 conversations, sentiment analysis while ensure you’re not blind to those interactions and potential areas for organizational improvement.
2. Forums and discussions groups
People aren’t always upfront with support agents about their experiences but will share their experiences (especially bad ones) on community forums. This makes sentiment analysis an important tool for seeking out customer service opportunities and even initiating case opens. By monitoring discussions for signs of product confusion, service dissatisfaction, or frustration, you can reach out on the forums to direct customers to further assistance. It also shows other members that you’re proactive in your customer support efforts.
“Companies can get a lot more out of discussion forums by proactively monitoring without having people from a support organization reading things,” says Monnett. “Being able to do that automatically with sentiment analysis and SupportLogic tools enables support operations to focus more directly on their core means of engaging with customers while avoiding the blind spots.”
3. Peer-to-peer agent interactions
Agents are expected to help customers; it’s their job. Yet, when cases fall through the cracks, it’s often because they weren’t handled with enough urgency or something was missed in translation. Sentiment analysis helps prevent both situations as it can signal and flag cases for special treatment and keep agents from having to go back through hundreds of cases for context.
4. Self-service knowledge base recommendations
People appreciate the ability to access support docs to solve their own problems. Does your tutorial or article base have what they are looking for? By using sentiment analysis to scan and measure their article search queries, you can discover where they are in the support process. You’ll also see if they are frustrated, so you can present them with the right article or send them directly to customer support.
These are just a few examples of companies looking toward social media platforms to see what their customers are saying. Anywhere text is used to communicate, there’s a chance to deploy sentiment analysis technology.
Value beyond handling customer cases
Making your customers happy is the ultimate goal, but it’s not the only reason to implement this type of AI-based tech.
Product improvements
Data captured through sentiment analysis is a treasure trove of insights that can be applied to every aspect of the business, including billing, marketing, and product development. If the analysis shows trends in how customers feel about a product, for example, it could be addressed in the next iteration or update. There’s no need to wait for a customer feedback survey or formal market research panel.
For busy developers with hundreds of feature requests, sentiment analysis can also help prioritize updates if they are tied to especially high negative sentiment. The engineering team can build features that lead to higher customer satisfaction and an ROI tied to KPIs instead of making changes based on theories.
Better work environment
Sentiment analysis provides real-time feedback to help teams anticipate needs. We know that B2B SaaS support centers can be high volume or high velocity, and in these scenarios, every minute counts. With real-time insight into how a customer feels or the ability to predict what they may feel in the near future, support agents can adjust interactions to mitigate frustration while shortening the feedback loop. A minute saved on hundreds of cases a day leads to a very efficient operation overall.
We also can’t forget that happy customers mean less stress for agents. With the insights gained from sentiment analysis, support teams will receive improved and applicable training based on data on the ways to deal with specific cases. This alone can reduce employee churn based on better experiences and a feeling that what they do matters.
Increased revenue
Finally, happy customers will buy more, even if they originally entered the interaction frustrated. By using sentiment analysis to gauge a customer’s feelings and experience, agents can know when and if it’s appropriate to also upsell. They don’t have to just solve a problem.
Measuring sentiment helps provide insights into when a customer is ready to take the plunge on upgrades or add-ons. It makes selling truly personal and lets your support agents do more than field angry calls; they can become solution consultants who add to your bottom line.
Leverage sentiment analysis to add value across your entire organization
While this technology can empower agents to address issues, this is just the beginning of what sentiment analysis can do for your brand. Consider how it helped 8×8, which used SupportLogic’s sentiment analysis technology to scan 100% of their 20,000 monthly support cases to predict 90% more cases that were close to escalating.
Would you like to have more insight into customer cases before they hit a breaking point? Take a guided product tour to see the difference sentiment analysis can make in your workflows.
Don’t miss out
Want the latest B2B Support, AI and ML blogs delivered straight to your inbox?